About this course
This course covers the data science perspective on the introductory concepts in machine learning, with a focus on making predictions. It covers how to build different models such as K-NN, decision trees and linear classifiers as well as important concepts such as data splitting and fundamental rules and laws. In addition, this course will teach you how to evaluate models properly and question their validity all while streamlining the process with pipelines.
About the program
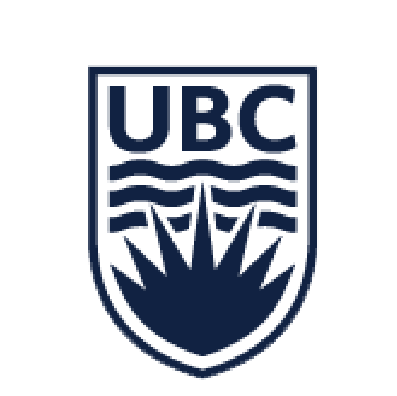
The University of British Columbia (UBC) is a comprehensive research-intensive university, consistently ranked among the 40 best universities in the world. The Key Capabilities in Data Science program was launched in September 2020 and is developed and taught by many of the same instructors as the UBC Master of Data Science program.